Philip Tetlock
Solomon Labs
3720 Walnut St, Room C8
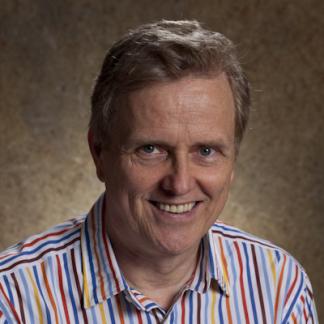
Decision Processes; Social and Cultural Psychology
Research Synopsis
- Learning from experience: How do experts think about possible pasts (historical counterfactuals) and probable futures (conditional forecasts)? And how do experts respond to confirmation/disconfirmation of expectations?
- Designing accountability systems: How do people cope with various types of accountability pressures and demands in their social world? When does accountability promote mindless conformity? Defensive bolstering of prior positions? Thoughtful self-critical analysis?
- De-biasing judgment and choice. How can organization structure incentives and accountability procedures to check common cognitive biases such as belief perseverance and over-confidence? What adverse side effects can such de-biasing efforts have on quality of decision-making?
Professor Philip Tetlock will not be considering new graduate students for admission for Fall 2025.
PSYC 2750 Political Psychology
PSYC 6000 (proseminar) Social Psychology
BA, University of British Columbia;
PhD, Psychology, Yale University
Books and Edited Volumes:
Tetlock, P. E. (2005, first edition; 2017, second edition). Expert political judgment: How good is it? How can we know? Princeton, NJ: Princeton University Press.
Tetlock, P. E., & Gardner, D. (2015). Superforecasting: The Art and Science of Prediction. New York, NY: Crown.
Journal Articles and Book Chapters:
Tetlock, P. E. & Gill, D. J. (2025). Prediction and retrodiction. American Historical Review.
Tetlock, P. E. (2025). Tracking forecasting accuracy of geopolitical schools of thought and causes of their predictive successes and failures. Critical Review.
Schoenegger, P., Tuminauskaite, I., Park, P. S., Valdece, S., Bastos, R. & Tetlock, P. E. (2025) Wisdom of the silicon crowd: LLM ensemble prediction capabilities rival human crowd accuracy. Science Advances. ISSN 2375-2548.
Schoenegger, Coombs, S., Karger, E. & Tetlock, P.E. (2025). AI-Augmented predictions: LLM assistants improve human forecasting accuracy. Association for Computing Machinery (ACM): Transactions on interactive intelligent systems.
Karger, E. Bastani, H. Yueh-Han, Jacobs, Z. Halawi, D. Zhang, F. & Tetlock, P. E. (2025). ForecastBench: A dynamic benchmark of AI forecasting capabilities. arXiv:2409.19839v1 [cs.LG] 30 Sep 2024.
Karger, E., Jacobs, Z., Rosenberg, J. & Tetlock, P. E. (2025). Subjective-probability forecasts of existential risk: Initial Results from a hybrid persuasion-forecasting tournament. International Journal of Forecasting.
Tetlock, P. E. (2024). Robert Jervis: The consummate fox. In Richard Immerman, Stacie Goddard, and Diane Labrosse (eds.), The Jervis Effect. Columbia University Press.
Atanasov, P., Witkowski, J., Mellers, B., & Tetlock, P. (2024). Crowd prediction systems: Markets, polls, and elite forecasters. International Journal of Forecasting. doi:10.1016/j.ijforecast.2023.12.009
Tetlock, P. E., Karvetski, C., Satopää, V. A., & Chen, K. (2024). Long-range subjective-probability forecasts of slow-motion variables in world politics: Exploring limits on expert judgment. Futures and Foresight Science, 6(1), e157. doi:10.1002/ffo2.157
Tetlock, P. E., Karvetski, C., Satopää, V. A., & Chen, K. (2024). Exploring the limits on Meliorism: A commentary on Tetlock et al. (2023). Futures and Foresight Science, 6(1), e173. doi:10.1002/ffo2.173
Clark, C. J., Isch, C., Connor, P., & Tetlock, P. E. (2024). Assume a can opener. Behavioral and Brain Sciences, 47, e36. doi:10.1017/S0140525X2300239X
Powell, B., Satopää, V. A., MacKay, N., & Tetlock, P. E. (2024). Skew-adjusted extremized-mean: A simple method for identifying and learning from contrarian minorities in groups of forecasters. Decision, 11(1), 173-193. doi:10.1037/dec0000191
Mitchell, G., & Tetlock, P. E. (2024). Stretching the limits of science: Was the implicit-racism debate a 'bridge too far' for social psychology? In J. A. Krosnick, T. H. Stark & A. L. Scott (Eds.), The Cambridge handbook of implicit bias and racism (pp. 147–165). Cambridge: Cambridge University Press.
Marcoci, A., Webb, M. E., Rowe, L, Barnett, A., Primoratz, T., Kruger, A., Karvetski, C. W., Stone, B., Diamond, M. L., van Gelder, T., Tetlock, P. E., & Dennis, S. (2023). Validating a forced-choice method for eliciting quality-of-reasoning. Behavioral Research Methods. doi:10.3758/s13428-023-02234-x
Clark, C. J., Jussim, L., Frey, K., Stevens, S. T., al-Gharbi, M., Aquino, K., Bailey, J. M.,Barbaro, N., Baumeister, R. F., Bleske-Rechek, A., Buss, D., Ceci, S., Del Giudice, M.,Ditto, P. H., Forgas, J. P., Geary, D. C., Geher, G., Haider, S., Honeycutt, N., Joshi, H.,Krylov, A. I., Loftus, E., Loury, G., Lu, L., Macy, M., Martin, C. C., McWhorter, J., Miller, G., Paresky, P., Pinker, S., Reilly, W., Salmon, C., Stewart-Williams, S., Tetlock, P. E., Williams, W. M., Wilson, A. E., Winegard, B. M., Yancey, G., & von Hippel, W. (2023). Prosocial motives underlie scientific censorship by scientists: A perspective and research agenda. Proceedings of the National Academy of Sciences, 120(48), e2301642120. doi:10.1073/pnas.2301642120
Clark, C. J., Graso M., Redstone, I., & Tetlock, P. E. (2023). Harm Hypervigilance in Public Reactions to Scientific Evidence. Psychological Science, 34(7), 834-848. doi:10.1177/09567976231168777
Mellers, B. A., McCoy, J. P., Lu, L., & Tetlock, P. E. (2023). Human and algorithmic predictions in geopolitical forecasting: Quantifying uncertainty in hard-to-quantify domains. Perspectives on Psychological Science, 0(0). doi:10.1177/17456916231185339
Grossmann, I., Feinberg, M., Parker, D. C., Christakis, N. A., Tetlock, P. E. & Cunningham, W. A. (2023). AI and the transformation of social science research. Science, 380(6650), 1108-1109. doi:10.1126/science.adi1778
Clark, C. J., & Tetlock, P. E. (2023). Adversarial collaboration: The next science reform. In C. L. Frisby, R. E. Redding, W. T. O’Donohue, & S. O. Lilienfeld (Eds.), Ideological and political bias in psychology: Nature, scope, and solutions (pp. 905-927). Cham: Springer International Publishing.
Tetlock, P. E., Lu, Y., & Mellers, B. A. (2023). False dichotomy alert: Improving subjective-probability estimates vs. raising awareness of systemic risk. International Journal of Forecasting, 39(2), 1021-1025. doi:10.1016/j.ijforecast.2022.02.008
Satopää, V. A., Salikhov, M., Tetlock, P. E., & Mellers, B. (2023). Decomposing the effects of crowd-wisdom aggregators: The bias–information–noise (BIN) model. International Journal of Forecasting, 39(1), 470-485. doi:10.1016/j.ijforecast.2021.12.010