Anna Schapiro
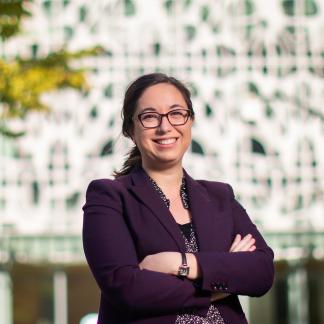
Memory; Learning; Neural network modeling; Sleep; Consolidation; Hippocampal-cortical interactions
Research Synopsis
Some moments in our lives provide information that is useful in itself, but other information is most meaningful when combined across many instances, as we come to understand the regularities in the world. How do we extract such structured knowledge from our environment? Answering this question requires an understanding of the initial acquisition of this information as well as its stabilization and integration with existing knowledge structures over time and with sleep. Our research combines neural network modeling and empirical methods (fMRI, EEG, behavior) to uncover learning algorithms and principles of how memories of regularities in the environment come to be represented throughout the brain.
Professor Anna Schapiro will be considering new graduate students for admission for Fall 2025.
B.S., Symbolic Systems, Stanford University
Ph.D., Psychology and Neuroscience, Princeton University
Siefert, E.M., Uppuluri, S., Mu. J., Tandoc, M.C., Antony, J.W., & Schapiro, A.C. (2024). Memory reactivation during sleep does not act holistically on object memory. Journal of Neuroscience.
Sucevic, J. & Schapiro, A.C. (2023). A neural network model of hippocampal contributions to category learning. eLife.Zhou, Z., Singh, D., Tandoc, M.C., Schapiro, A.C. (2023). Building integrated representations through interleaved learning. Journal of Experimental Psychology: General.
Solomon, S.H. & Schapiro, A.C. (2023) Structure shapes the representation of a novel category. Journal of Experimental Psychology: Learning, Memory, and Cognition.
Singh, D., Norman, K.A., Schapiro, A.C. (2022). A model of autonomous interactions between hippocampus and neocortex driving sleep-dependent memory consolidation. Proceedings of the National Academy of Sciences.
Schapiro, A.C., McDevitt, E.A., Rogers, T.T., Mednick, S.C., & Norman, K.A. (2018). Human hippocampal replay during rest prioritizes weakly-learned information and predicts memory performance. Nature Communications.
Honey, C.J., Newman, E.L., & Schapiro, A.C. (2017). Switching between internal and external modes: a multi-scale learning principle. Network Neuroscience.
Schapiro, A.C., Turk-Browne, N.B., Botvinick, M.M., & Norman, K.A. (2017). Complementary learning systems within the hippocampus: A neural network modelling approach to reconciling episodic memory with statistical learning. Philosophical Transactions of the Royal Society B.
Schapiro, A.C., Gregory, E., Landau, B., McCloskey, M., Turk-Browne, N.B. (2014). The necessity of the medial temporal lobe for statistical learning. Journal of Cognitive Neuroscience.
Schapiro, A.C., Rogers, T.T., Cordova, N.I., Turk-Browne, N.B., & Botvinick, M.M. (2013). Neural representations of events arise from temporal community structure. Nature Neuroscience.
Schapiro, A.C., Kustner, L.V., & Turk-Browne, N.B. (2012). Shaping of object representations in the human medial temporal lobe based on temporal regularities. Current Biology.
Advisees
Alexander (Alex) Gordienko [Psychology Graduate Student]
Dhairyya Singh [Psychology Graduate Student]
Marlie Tandoc [Psychology Graduate Student]
Zhenglong Zhou [Psychology Graduate Student]